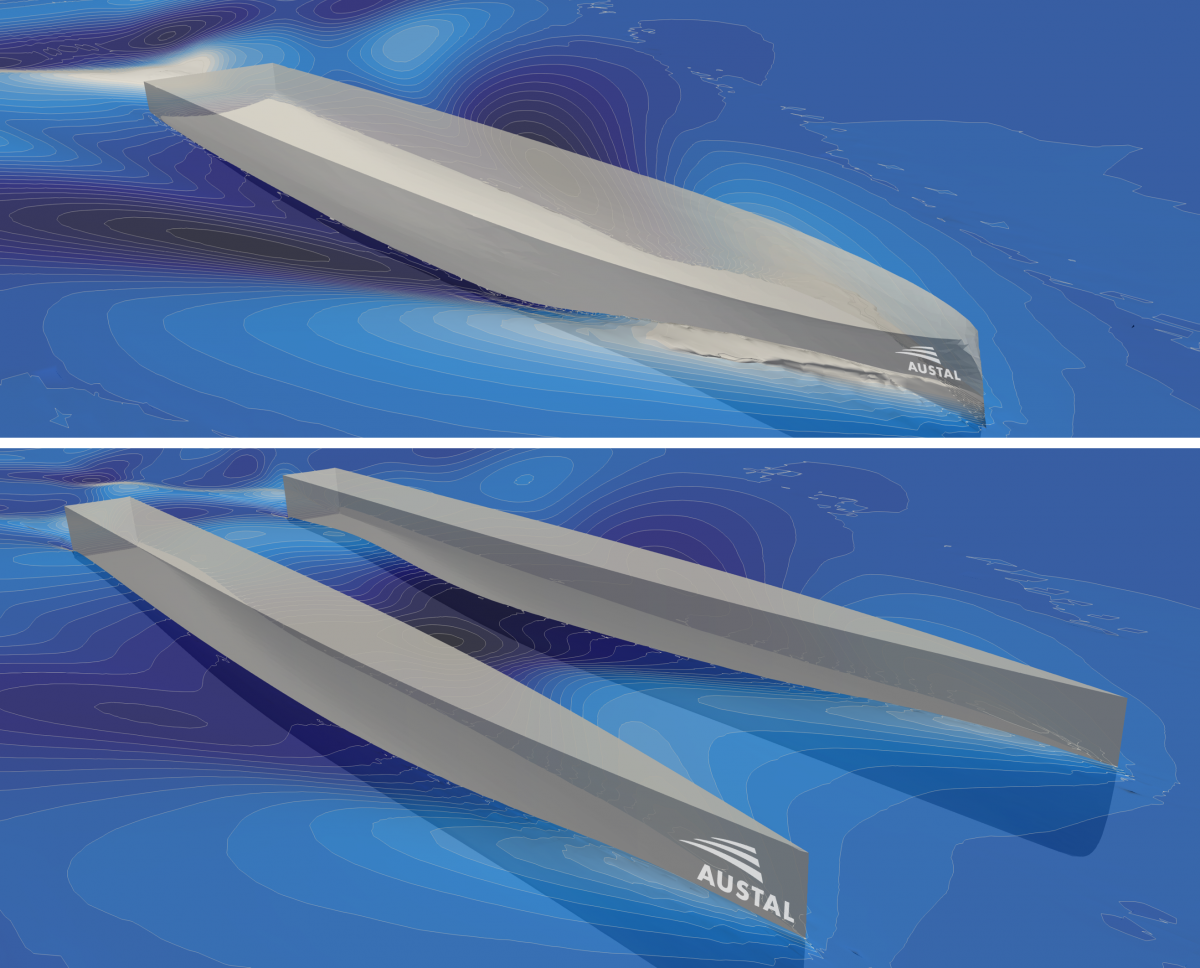
Austal, an Australian shipbuilder, has recently developed an AI toolset called DeepMorpher. This new technology has enabled the company’s designers to streamline the complex hull optimisation process, resulting in a significant reduction in resource requirements.
Aware that market dynamics favour companies that can quickly adapt to emerging trends such as autonomy, decarbonisation and new naval capabilities, Austal Australia developed DeepMorpher software, with the aim of revolutionising maritime engineering through the application of AI.
A spokesperson for Austal indicated that Deepmorpher is already seeing wide usage in the company’s design process. “DeepMorpher has been developed to augment and streamline Austal’s existing workflows for the optimisation of products across our entire portfolio, and we are currently using this new capability to optimise monohull and multi-hull concepts in both the defence and commercial markets,” they said.
According to Max Haase, a development hydrodynamics specialist at Austal Australia and co-author of a recently published research article titled ‘DeepMorpher: Deep learning-based design space dimensionality reduction for shape optimisation‘, the new technology enables the company to explore and enhance various hull forms that are not typically included in its product range in a more efficient manner.
How well do you really know your competitors?
Access the most comprehensive Company Profiles on the market, powered by GlobalData. Save hours of research. Gain competitive edge.
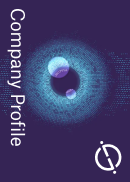
Thank you!
Your download email will arrive shortly
Not ready to buy yet? Download a free sample
We are confident about the unique quality of our Company Profiles. However, we want you to make the most beneficial decision for your business, so we offer a free sample that you can download by submitting the below form
By GlobalDataAccording to Haas, the implementation of this technology would decrease the need for specialised knowledge and allow for a stronger emphasis on achieving optimal results in accordance with customer requirements.
See Also:
The implementation of AI and machine learning has revolutionised the optimisation process, providing customers with a wider range of options in a shorter amount of time. According to a Haase, the time frame for hull form optimisation in conjunction with computational fluid dynamics can be reduced ‘by an order of magnitude’ with the support of high-performance computing provider DUG Technology.
The efficacy of the Deepmorpher AI software was demonstrated for the Journal of Engineering Design through a simulation-driven design optimisation approach that employed several baseline templates and multi-objective Bayesian optimisation to identify optimal designs.