As artificial intelligence (AI) becomes more pervasive and embedded in life-changing decisions, the need for transparency has intensified. There have been plenty of high-profile cases in recent years where AI has contributed to bias and discrimination, with the use of facial recognition for policing just one example. There is a high probability of a shift from loose self-regulation to government involvement in AI over the next couple of years. In turn, Big Tech is increasingly using AI to solve the privacy and bias problems that the technology itself created.
Technology Trends
Listed below are the key technology trends impacting the AI theme, as identified by GlobalData.
Explainable AI (XAI)
AI is increasingly involved in life-changing decisions, such as welfare payments, mortgage approvals, and medical diagnoses. Consequently, transparency and explainability have become essential. Controversies surrounding bias in AI models have received a lot of attention, prompting companies to update their internal AI development guidelines to build trust. XAI alone will not be enough to mitigate bias in the data, as it will only enable companies to identify potential discrimination. It will be up to businesses to correct their models as needed.
XAI and responsible AI have emerged as a top priority for most companies involved with AI such as Google and defence giant Lockheed Martin.
Federated learning
AI as a solution to the privacy problem that the technology itself created might sound counterintuitive. The approach, introduced by Google in 2017, aims to use the rich datasets that are available on local devices, such as smartphones, while at the same time protecting sensitive data. Put simply, a device downloads the current model from the cloud, improves it by learning from data stored in its memory, and then updates the model based on the localised data.
Gboard is the most well-known app that uses federated learning. Google’s keyboard app suggests words or phrases based on data from previous actions. In the healthcare industry, federated learning allows different organisations to collaborate on a model that will improve patient care without sharing sensitive data.
How well do you really know your competitors?
Access the most comprehensive Company Profiles on the market, powered by GlobalData. Save hours of research. Gain competitive edge.
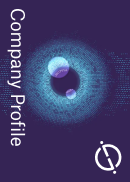
Thank you!
Your download email will arrive shortly
Not ready to buy yet? Download a free sample
We are confident about the unique quality of our Company Profiles. However, we want you to make the most beneficial decision for your business, so we offer a free sample that you can download by submitting the below form
By GlobalDataFacial recognition
In June 2020, Amazon, IBM, and Microsoft all announced that they would no longer sell facial recognition (FR) tools, either to law enforcement or in general. While Amazon put a one-year moratorium on the use of FR by law enforcement, Microsoft said it would not sell FR to police departments until there was a national law to regulate the technology. In turn, IBM announced that it would no longer offer general-purpose FR or analysis software.
Increased scrutiny following the killing of George Floyd and the subsequent anti-racist protest have encouraged Big Tech companies to distance themselves from FR, but ethical concerns and examples of bias have been prevalent for years.
Automated machine learning (AutoML)
AutoML aims to reduce the amount of human input and energy needed to apply ML to real-world problems. It does this by automating the time-consuming, iterative aspects of ML model development, allowing developers and data scientists with limited ML expertise to use ML models and techniques. It also reduces human error and bias in ML models.
Fraud detection, pricing, and sales management are common application areas of AutoML. Google Cloud AutoML, DataRobot, and H20.ai are examples of leading AutoML software solutions.
Doing more with less
In an interview from 2019, Jeff Dean, head of Google AI, said: “We want to build systems that can generalise to a new task. Being able to do things with much less data and with much less computation is going to be interesting and important.” Most agree that the regulatory and business advantages of learning from fewer data points are significant.
Rana el Kaliouby, CEO and co-founder of emotion recognition start-up Affecta, has stated that data synthesis methods will allow companies to take data that has been collected and synthesise it to create new data. For example, a video of someone driving a car can be used to create new scenarios, such as a driver turning their head or texting.
AIoT on the edge
One of the main areas of convergence between disruptive themes is AI and IoT. This combination has given rise to AIoT – Artificial Intelligence of Things. Combining data collected by connected sensors and actuators with AI allows for reduced latency, increased privacy, and real-time intelligence at the edge.
Apple acquired Xnor.ai, which offered AI-enabled image recognition tools capable of functioning on low-power devices, in January 2020 to bolster its offering at the edge.
Quantum computing
Quantum computing is starting to come of age, with Google, Microsoft, and IBM leading the pack. AI applications could work much faster if backed by quantum computers. According to Dr. David Awschalom, professor of quantum information at the University of Chicago, the use of quantum computers could speed up many computational tasks that underline ML. They would enable faster calculations and allow for the development of more resource-efficient algorithms, while allowing AI to tackle more complex tasks.
IBM has been offering quantum cloud computing services to customers since 2016, and, in 2019, Microsoft (Azure Quantum) and Amazon (Quantum Solutions Lab) both announced that customers would potentially be able to test their experimental algorithms on multiple quantum computers.
Next-generation chips
The emphasis in chip design has shifted from a race to place more transistors onto a square millimetre of silicon to a focus on building microprocessors as systems, made up of multiple components, each of which performs a specialised task. Over the next few years, computers will increasingly mimic how the human brain stores and processes information. This is where neuromorphic computing and neuromorphic chips come in.
Neuromorphic chips are well-suited to handling large amounts of data and powering deep learning applications that use neural networks while consuming less energy than conventional processors. IBM (with TrueNorth) and Intel (with Loihi) currently lead the pack in R&D but face competition from well-established players such as Qualcomm, HPE, and Samsung, and well-funded start-ups like Graphcore and Cambricon.
This is an edited extract from the Artificial Intelligence, 2020 Update – Thematic Research report produced by GlobalData Thematic Research.